2025 Vol. 52, No. 4
Cover story: Han T, Ye J, Yan L S, et al. Adaptive mesh partitioning for graph attention Transformer networks[J]. Opto-Electron Eng, 2025, 52(4): 250058
In modern scientific research and engineering applications, complex geometric models and physical phenomena are often modeled using partial differential equations (PDEs) and solved using numerical methods such as the finite element method (FEM) or finite volume method (FVM).Adaptive mesh refinement (AMR) can significantly alleviate the conflict between computational accuracy and efficiency and has been applied in many fields such as solid mechanics, fluid dynamics, aerospace, and acoustics.
The Information Photon and Communication Research Team of the School of Information Science and Technology at Southwest Jiaotong University has proposed an adaptive mesh partitioning method based on graph neural networks, called the GATv2-Transformer Fusion Network (GTF-Net).This method transforms the adaptive mesh refinement problem into a node classification task, where each mesh node in the initial grid is treated as a sample to be classified.
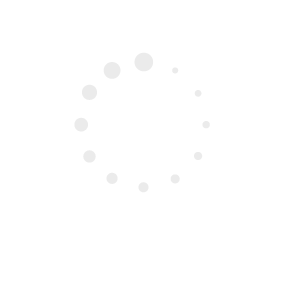
-
{{article.year}}, {{article.volume}}({{article.issue}}): {{article.fpage | processPage:article.lpage:6}}. doi: {{article.doi}}{{article.articleStateNameEn}}, Published online {{article.preferredDate | date:'dd MMMM yyyy'}}, doi: {{article.doi}}{{article.articleStateNameEn}}, Accepted Date {{article.acceptedDate | date:'dd MMMM yyyy'}}CSTR: {{article.cstr}}
-
{{article.year}}, {{article.volume}}({{article.issue}}): {{article.fpage | processPage:article.lpage:6}}. doi: {{article.doi}}{{article.articleStateNameEn}}, Published online {{article.preferredDate | date:'dd MMMM yyyy'}}, doi: {{article.doi}}{{article.articleStateNameEn}}, Accepted Date {{article.acceptedDate | date:'dd MMMM yyyy'}}CSTR: {{article.cstr}}